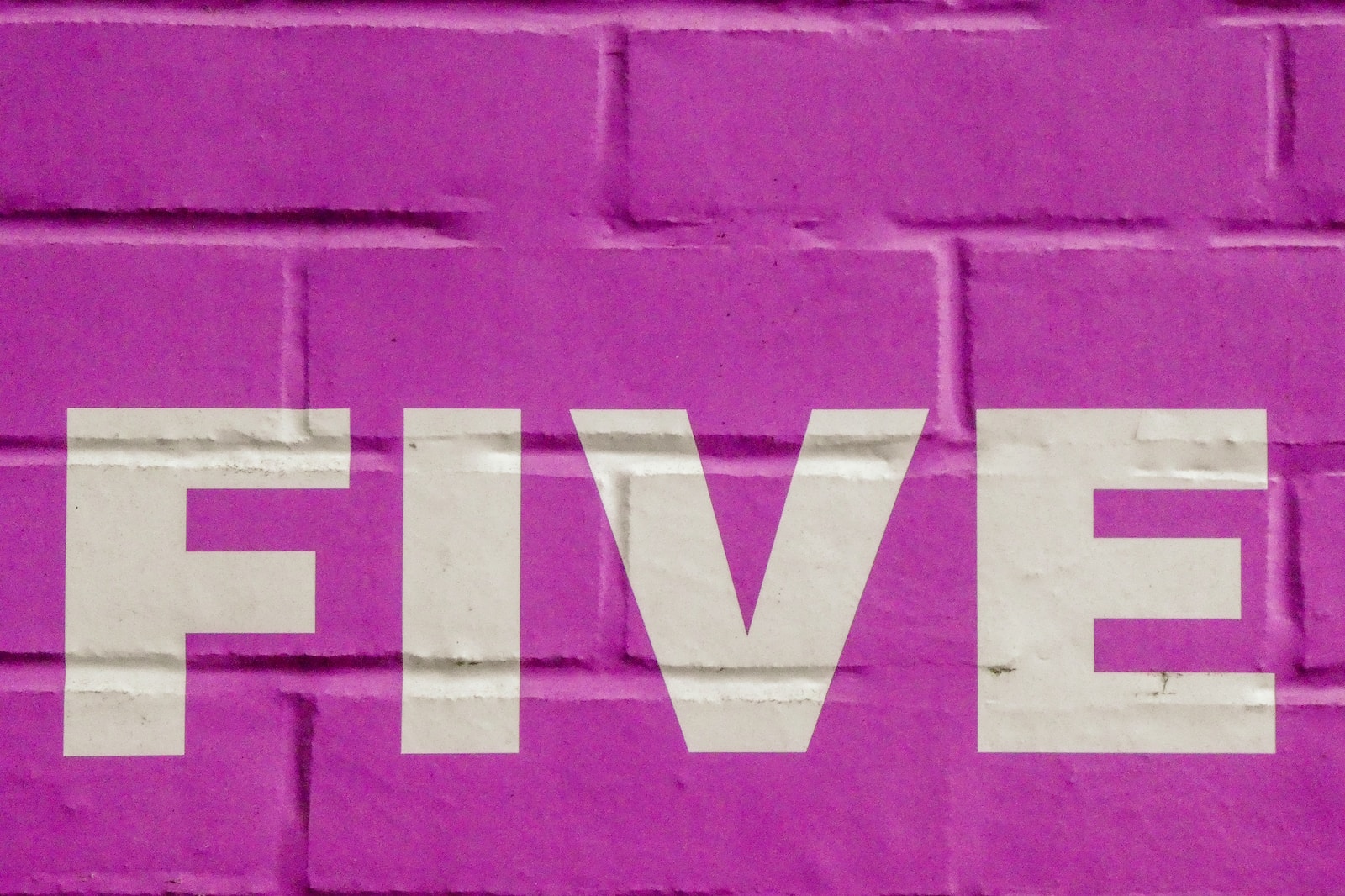
If you are in an IT role responding to data prep needs from the business, you have likely seen some variation of an analyst emerge at your company. Viewed as the most plausible answer to a shortage of Ph.D. data scientists, analysts work with data but may not have a formal education in business intelligence and statistics, as this article in Forbes notes. But as 451 Research recently reported, “the role might need some careful handling in order to live up to its full potential.”
According to this recent Gartner report, “Analytics users spend the majority of their time either preparing their data for analysis or waiting for data to be prepared for them”. Ph.D. analysts have a strong DIY programmer mindset and can comfortably spend the requisite 79% of analytic project time (according to Crowdflower) doing complex data preparation themselves. In contrast, analysts work in visual environments and still rely heavily on an IT organization for anything outside of that paradigm – including accessing new data sources, transforming data and generally preparing data for analysis.
With huge growth expected in the number of analysts, IT managers need a more systematic and scalable way to serve the ever-increasing data preparation needs of analysts. Otherwise, you will likely end up needing to add more IT staff, spend your days doing repetitive and mundane data preparation tasks – and more importantly, put your data integrity and quality of business decision-making at risk. For more on self-service data prep, read the Gartner Research: Market Guide to Self-Service Data Preparation.
The following are five ways you can analysts to prepare data using a self-service model, set them up to build predictive models that make your business more competitive and maintain data governance – all on your own terms.
1) Provide easy access to new and varied data sources
Building new and innovative predictive models often requires access to myriad data sources – and these sources are continually evolving. Many analysts are working with structured CRM data and Excel data, plus semi-structured data such as web log data and new and emerging data sources such as twitter for sentiment analysis. Instead of hiring new ETL developers to write custom code each time a citizen data scientist wants to access a new data source, use a data integration tool that uses a drag and drop paradigm, includes pre-built steps and provides the ability to inspect and analyze data along the pipeline. We have seen some organizations go from 1,500 custom-coded ETL transformations to fewer than 10 by taking a visual and templated approach. If you estimate a cost of $1,000 per custom-coded ETL transformation, that would be an enormous savings.
2) Provide the guardrails for proper data delivery and governance
This is critical. IT needs to provide the guardrails for end-consumers of data to do their own data preparation. This begins with establishing consistent, end-to-end visibility into activities across the data pipeline, from ingestion to preparation to analytics. Data pipelines must be architected with full knowledge of underlying systems and their constraints. Further, the semantics and auditability of source data must be preserved in order to provide a trusted and accurate foundation for a self-service environment. Secure, role-based access to different data preparation activities is also a must.
3) Allow integration between legacy and emerging data sources together
To help analysts build even more predictive models, provide them with the tools to blend old historical, data warehouse data with new sometimes big or “dark” data that hasn’t been tapped. These data sources include GPS data, sentiment data, mobile app data, etc. Even at a basic level, giving a citizen data scientist an intuitive way to blend and join different data sets or enrich their data sets with demographic data, location data, clickstream data or purchase data, can unlock tremendous value.
Ruckus Wireless is a good example of this. They were able to shed light on dark data from their customer’s Wi-Fi networks, by leveraging massive JSON and XML data to provide granular visibility into network utilization and performance in a way their customers had never seen.
4) Use a unified platform that’s agnostic relative to data and predictive models
To reiterate, analysts are not programmers – and they don’t want to learn a number of new data preparation tools. Look for a platform that includes both data integration and analytics in one intuitive platform – and one that is agnostic with regard to data type, source and predictive analytics packages. Pentaho also offers a Data Science Pack that allows users to orchestrate and run third party models inside of our software (i.e. from R, Python, or Weka). You want a future-proof platform that can grow and adapt with your organization.
5) Let analysts build data marts on-demand
One approach is to provision a web application that allows analysts to build data marts on demand, kicking off dynamic data blending from multiple sources and refinement when the user requests it. The user access controls can be configured based on security level and show only the back-end databases they have access to in the enterprise. This enables true self-service data access and prevents the end-user from having to go back to IT every time they want access to a new data mart or other data source.
Pentaho has several customers at various stages of delivering this data mart on-demand concept, including FINRA, the largest independent regulator for all securities firms doing business in the United States. By leveraging a 7 petabyte data lake, end-users can create data sets on the fly for detecting fraudulent activities, without needing to go back to IT every time they need to modify the underlying data.
In conclusion, analysts represent an enormous opportunity for organizations to become more data-driven and gain significant competitive advantages through predictive analytics – without needing to hire an army of Ph.D. data scientists. But they are a unique class of analysts that requires specialized tools and enablement. If you provide them with the right tools, capabilities and most importantly self-service data preparation with the right overarching governance, you can really scale the analytics initiatives at your company.
Want to learn more about empowering analysts with the tools they need to succeed? See how Pentaho makes data preparation easy.
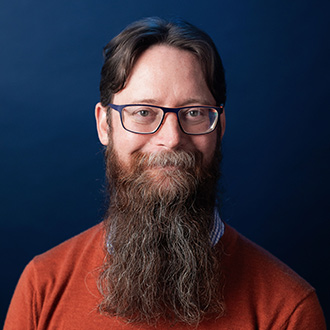
Zachary Zeus
Zachary Zeus is the Founder of BizCubed. He provides the business with more than 20 years' engineering experience and a solid background in providing large financial services with data capability. He maintains a passion for providing engineering solutions to real world problems, lending his considerable experience to enabling people to make better data driven decisions.